Based on a presentation by Chris Lindner – Manager, Product Science at Indeed, watch the full presentation here.
Note: This talk was presented in February, and the job market has changed quite a bit amidst the COVID-19 pandemic. While not specific to jobs in data science, Chris recommends checking out the data Indeed economists have released from the Indeed Hiring Lab for updated hiring data.
At #DSSATX (overall event recap here), we soaked up a data-driven talk on data science hiring patterns and trends by a guy who would know: Chris Lindner, manager of product science for Indeed, the world’s number one job site with 25 million active job postings, 250 million unique visitors per month, and 150 million posted resumes. That’s a lot of data to draw conclusions from about job statistics at scale.
Chris opened by saying “I want to do a little data sciencing on data science and see what exactly we can learn about our field.” And so we do!
DATA SCIENCE JOB POSTINGS
Since 2013, data science job postings have increased by 256 percent—but even crazier: growth is still continuing. In 2018, there was a 31% increase in data science job postings compared to the previous year.
At the same time, people have heard good things about data science as a career, so we can also look at how many people are actually searching for these jobs. In 2018, there’s a 14 percent increase year-over-year in searches for data science jobs.
If you compare the 14 percent increase in job seekers to the 31 percent increase in job posting, it's clear supply is still well under demand.
WHAT IS A DATA SCIENTIST ROLE, REALLY?
Ask anybody with the data science title, what they do day-to-day, and you’ll find that they do wildly different things. Even though universities are now offering data science degrees, there's no consensus on what the heck it is or what should be taught in these courses.
In order to explore the data further, the Indeed team talked to employers and tried to understand a little better what they were looking for in a data scientist. They found that some companies are just renaming a lot of old jobs that have already existed—so business analyst-type jobs are being rebranded because “data scientist” is a sexy title even if they're not paying any differently or changing anything about what that role actually does. Other companies, on the other hand, are looking for a data scientist who has experience with machine learning, there's often advanced degrees involved, so that role is actually something distinct from older analyst roles.
But can we actually look at the data and even figure out if there are different groupings? If there are different job titles embedded in the monolith of data science? The Indeed team did that by looking at search queries from different individuals who are searching “data scientist” and comparing that to the other things those individuals are searching for to see if there are patterns in the data.
There are some very clear clusters into these four categories:
- Business Intelligence: analysts, analytics, BI developers, data analysts
- Machine learning: focus on artificial intelligence, Spark, and Big Data platform
- Statisticians: accounting, biostats, data mining
- Science roles: former or current academics looking for biology, biotech, physics, PhD work
This clustering maps to Indeed’s understanding of data scientists as well: under the umbrella of data science, there are data scientists and product scientists. Data scientists are probably a little closer to what you would call a machine learning engineer; like a lot of other companies, Indeed product scientists tend to be more generalist; there are separate analytics, BI analysts, and BI developers as well.
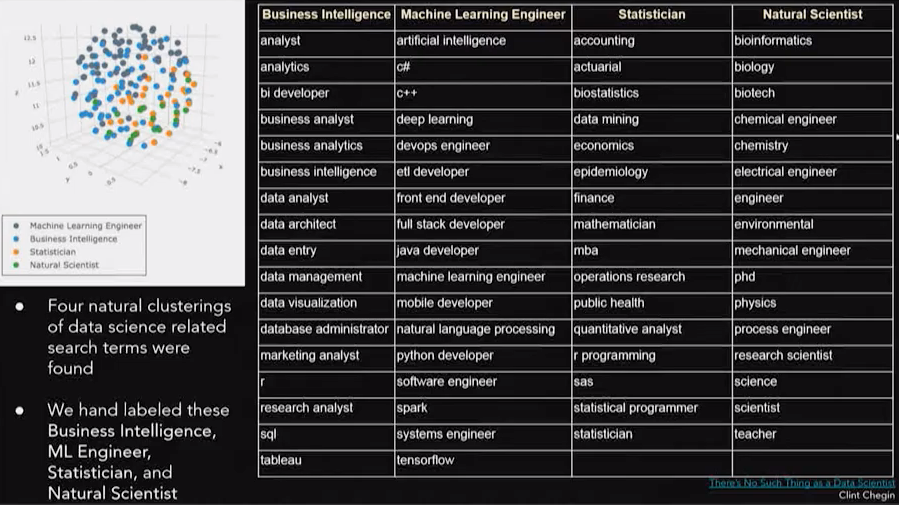
WHAT’S ON A DATA SCIENTIST’S RESUME?
So we've identified that there are different job titles and different roles in data science; but are there actually differences in their backgrounds?
The following observations are focused on five job titles: data scientist, machine learning engineer, software engineer, data analysts, or data engineer.
- Data scientists and machine learning engineers usually require advanced degrees, PhDs and master's degrees; 75% of folks with the data scientist title had a master's degree or higher and about 20% had PhDs.
- Software engineers, data analysts, and data engineers are more likely just to have bachelor's degrees.
- You also see that data engineers have the highest concentration of solely high school diploma earners, which Chris attributes to data engineers coming from very untraditional backgrounds. There is not a very straightforward path to becoming a data engineer right now, many are self-taught, whereas you see very little of that over in data scientist land and machine engineer roles.
What are these degrees in?
- In the data science role there is a lot of variance, different types of fields from the natural sciences, social sciences, engineering, and computer science.
- Machine learning engineers and especially software engineers are pretty heavily focused on computer science backgrounds and engineering backgrounds.
- In data analysts, you see a lot more business degrees.
- Data engineers tend to be all over the place, although a little bit more focused on engineering than data scientists.
TRENDS OVER TIME
Education level: in 2013, we were mostly seeing doctorates and masters for data scientists. But as time goes on there are a whole lot less PhDs, a dip in master's degrees, and the rise of the bachelor's degree data scientists. Nowadays, people with bachelor's degrees go straight out of school into data science roles.
This makes a lot of sense if you think about the employment gap discussed earlier, where companies are just not filling data science roles fast enough. To get more people, it makes sense to start looking at bachelor's degree holders to fill these roles.
A data science degree is a newer field of study, but more and more common: 15% of people have a degree in data science.
What you don't see is a lot of people leaving data science; it seems to be sort of the endpoint where once people get a data science title that they tend to keep that title.
A lot of software engineers are the ones that are becoming data engineers.
SPECIFICITY IN JOB DESCRIPTIONS
At Indeed, data scientists and product scientists are different job titles, even though product science is not an industry-standard term. In writing job descriptions, the team wondered if their nomenclature was reducing how attractive the job looked to job-seekers. So they ran an A/B test across cities between the titles data scientist and product scientist.
Basically the short answer is: once you change that name from product scientist to data scientist, data scientists are gonna get more clicks.
This is probably one of the reasons that a lot of companies are just choosing to use that generic data scientist job title: they know that's what people are looking for, people value that data scientist job title. But that is part of what’s creating ambiguity around the role.
Despite the data, Indeed sticks with the Product Scientist title even though it gets fewer clicks. Their reasoning is that the internal distinction is important: a product scientist does very different things than what a data scientist does. A product scientist is focused on the exploratory work, building models as exploratory work to see if a model is the right solution. If they identify a strong opportunity, it’s handed off to a data scientist, who is involved in implementing machine learning models, and tuning and tweaking those, making sure all the data sources are right—essentially doing the actual implementation.
Of course, like all data science roles, there's a lot of blurring there! There are product scientists who have production models and are maintaining those, or data scientists who do a lot more analytics and a lot less of the production work.
Data scientists, product scientists, data engineers, and many of the other job titles covered here continue to be imbalanced in the job market: the supply falls well short of the demand. This is ultimately good news for our data community!
--
For more, you can check out the following blog posts, which are the sources of much of the information presented above.
- There’s No Such Thing as a Data Scientist by Clint Chegin
- Does Your Job Title Matter? by Zhuying Xu
- Where Do Data Scientists Come From? by Chris Lindner
- Data Science - A Hot Job that Pays Well by Andrew Flowers
Follow us @DataSciSalon on twitter.